|
Large models have become a hot topic last year. Major manufacturers such as Google, Microsoft, OpenAI, etc. are actively developing and applying large model technology. These models have shown amazing capabilities in language understanding, image recognition, recommendation systems, etc., and have even surpassed human performance in some tasks. Maybe you have used it and marveled at its magic, or maybe you have not used it and listened to its legend. In any case, I understand a little bit, but it is difficult to explain clearly, how is it generated? Next, we will explain the principles of its generation and understand four large-model technical architectures for building AI applications. 1.
The generation principle of the large model First of all, what we need to understand is that the GPT large model is a natural language processing model based on deep learning, which is LLM. Knocking on the blackboard, LLM is Argentina WhatsApp Number a model for generating text, such as DALL·E. It and LLM are both branches of the multi-modal language model. Its working principle can be simply understood as "the law of learning language", and its generation The method is just to guess the probability of the next word based on the above.
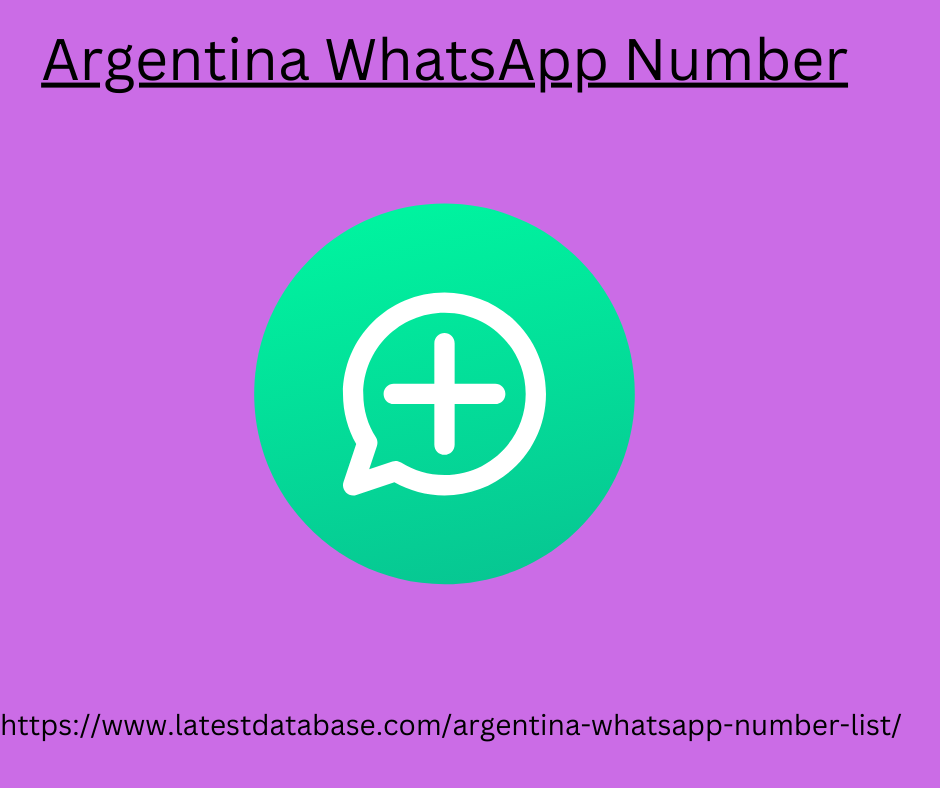
So why does it have so much knowledge? That's because during the model training process, the GPT model will read a large amount of text data and then learn the language patterns in these texts. This process can be compared to the way humans learn language. When we are babies, we learn the patterns of language by listening to our parents and those around us. How to define B-end products and B-end product manager methodology Compared with C-end products, the biggest feature of B-end.
|
|